End-to-end Ai implementation
The Ai economy extends beyond simple Ai model and automation utilization. This article show how to build Ai-driven solution for the Enterprise, end-to-end.
Aiforce & co. Editorial
9/25/20244 min read
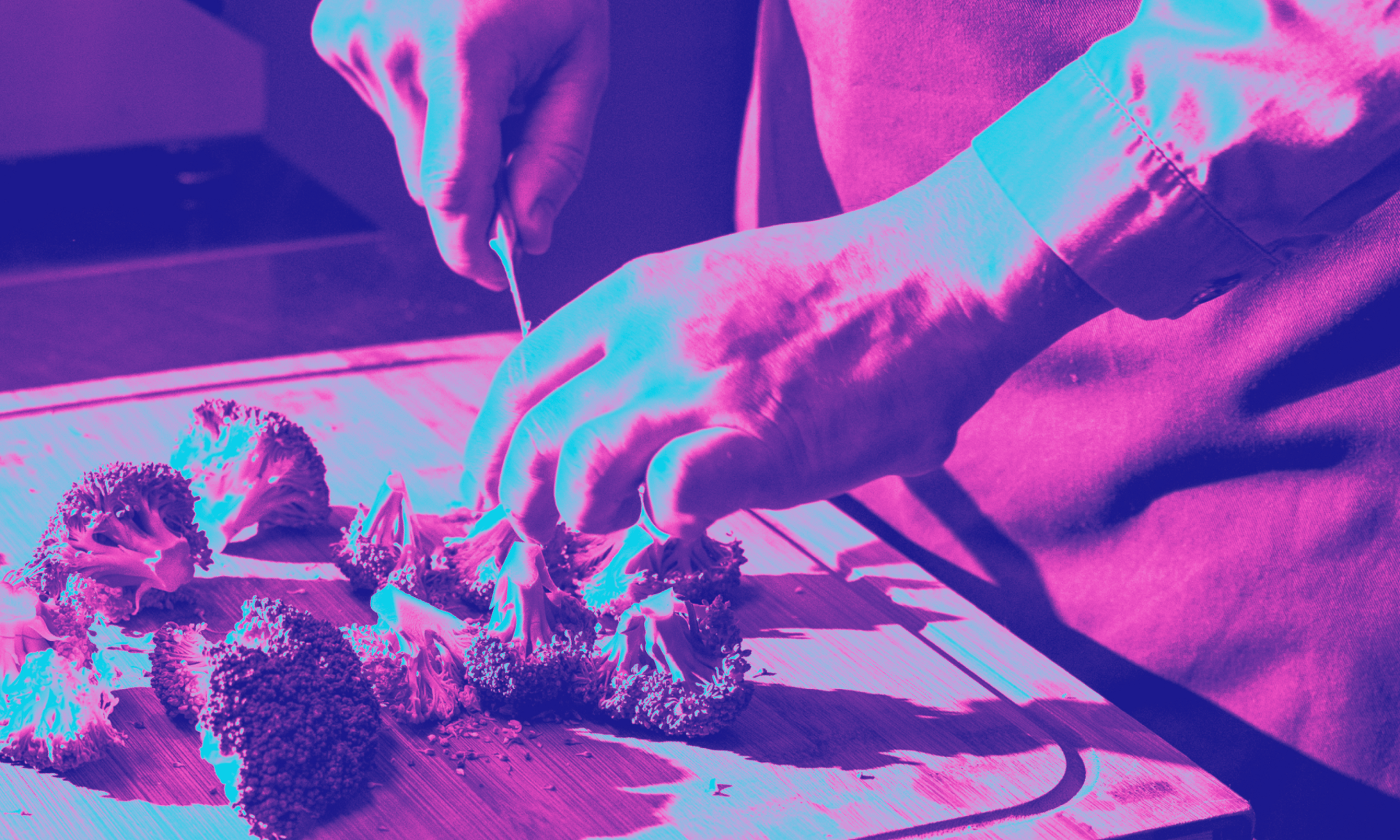
Full-Service AI Implementation: Bridging the Gap from POC to Enterprise Success
The promise of Artificial Intelligence (AI) has captivated enterprises globally. Many companies successfully complete Proof of Concepts (POCs) for AI initiatives, only to struggle with scaling these solutions and fully realizing their potential post-POC. What’s often missing is a clear roadmap for end-to-end AI implementation that goes beyond experimentation and moves into sustainable, enterprise-wide adoption. In this blog post, we will explore why enterprises often struggle post-POC and outline a full-service AI implementation approach that can ensure the success of AI initiatives beyond the proof-of-concept phase.
Why Enterprises Struggle Post-POC
Lack of Infrastructure Readiness POCs are often executed on small, isolated datasets, using basic infrastructure. Scaling AI across the organization requires robust, scalable data architectures, cloud infrastructure, and integration with existing systems. Enterprises often underestimate the infrastructure overhaul required for AI scalability.
Inadequate Change Management AI projects typically introduce new workflows, decision-making processes, and technologies. Many enterprises struggle to embed AI solutions into everyday operations because they overlook the cultural and organizational changes required for adoption. Teams may be resistant to AI-driven insights, or lack the training to use AI tools effectively. ####
Difficulty in Integrating AI with Business Processes AI initiatives that live in silos during the POC phase often fail to integrate smoothly with business processes when scaled. AI models must interface with existing systems such as CRM, ERP, and supply chain platforms, but this level of integration can be technically challenging and costly if not properly planned.
Unclear Metrics for Success While POCs are measured on short-term metrics—such as model accuracy or time savings—enterprises often lack the frameworks to assess long-term success in the implementation phase. Metrics such as ROI, impact on customer satisfaction, and operational efficiency need to be clearly defined and tracked over time.
Talent Gaps POCs are often driven by highly specialized AI experts or third-party consultants. However, operationalizing AI requires in-house talent capable of managing, maintaining, and optimizing the models over time. Many enterprises find themselves without the necessary skills to manage AI at scale.
The Full-Service AI Implementation
A full-service AI implementation approach ensures that AI models move beyond the POC and are integrated into the fabric of the enterprise. It addresses the unique challenges of scaling AI while delivering tangible business outcomes. Here’s a roadmap for full-service AI implementation:
Strategic Planning for Scale Successful AI implementation starts with a long-term vision, not just short-term experimentation. Enterprises should:
Define clear business objectives: AI should address strategic goals like increasing revenue, improving customer satisfaction, or enhancing operational efficiency. These objectives should be identified before moving into the scaling phase.
Create a roadmap: Outline the phases of implementation, including infrastructure upgrades, integration points, and team training. A roadmap ensures AI is deployed systematically rather than in ad hoc pockets across the organization.
Building Scalable AI Infrastructure To ensure AI can be used across the enterprise, companies need a flexible and scalable infrastructure:
Data Architecture: Establish a centralized data repository, either on-premise or in the cloud, to ensure models have access to high-quality, up-to-date data. Implement data pipelines to automate the collection, cleaning, and transformation of data.
Cloud and Hybrid Solutions: Leverage cloud platforms that offer the computational power needed for large-scale AI workloads. Hybrid models—combining cloud and on-premise resources—can be effective for enterprises with sensitive data or unique legacy systems.
Model Management: Implement ModelOps (similar to DevOps for AI), ensuring AI models can be deployed, monitored, and updated consistently across different departments.
End-to-End Integration with Business Processes AI solutions must be seamlessly integrated into business processes to provide real value:
API and Systems Integration: Build APIs that allow AI models to communicate with other enterprise systems like CRM, ERP, and supply chain platforms. This ensures that insights generated by AI are actionable within the current operational framework.
Automating Workflows: Use AI to automate decision-making within existing workflows. For example, AI can be integrated into the customer service workflow to triage and respond to inquiries, or into financial systems to automate risk assessments.
Change Management and Training Programs Even the most advanced AI solutions can fail if employees are not ready or willing to use them. A comprehensive change management plan ensures smooth adoption:
Leadership Buy-In: Senior executives must champion AI initiatives and communicate their importance to the broader organization. Leadership support can help overcome resistance to change and set the tone for AI’s role in strategic decision-making.
Upskilling Employees: Offer training programs to help employees at all levels understand how AI tools work, how they can improve their day-to-day tasks, and how to leverage AI insights. Developing AI fluency across the organization is key.
Cross-Functional Teams: AI is not just for the IT or data science departments. Create cross-functional teams with members from data science, IT, operations, and business units to ensure AI is implemented and maintained with broad business knowledge. ####
Clear KPIs and Success Metrics Defining success metrics is crucial for measuring the long-term impact of AI implementation:
ROI Calculation: Track the return on investment by comparing the cost of AI implementation against the financial gains it generates, such as increased revenue or reduced operational costs.
Operational Metrics: Measure efficiency gains, such as time savings from automated tasks, improvements in product quality, or reduction in manual errors.
Customer Impact: Track customer satisfaction improvements through AI-driven personalization, faster response times, or more accurate product recommendations.
A Full-Service AI Implementation: Case Study
Consider the example of a global retailer that completed an AI POC for personalized product recommendations. Post-POC, the retailer struggled to scale the AI model across its various markets due to infrastructure limitations and siloed data. By adopting a full-service AI implementation approach, they were able to:
Strategize for Scale: They developed a comprehensive roadmap that included setting up a centralized cloud-based data architecture and implementing robust APIs to connect the AI model with their e-commerce platform.
Integrate Across Channels: The AI recommendations engine was integrated across all customer touchpoints, including the website, mobile app, and physical stores. This ensured a seamless customer experience.
Train Employees: Their marketing and operations teams were trained to interpret AI-driven insights, which led to more targeted marketing campaigns and optimized inventory management.
Measure Success: The retailer tracked key performance metrics, including a 20% increase in sales from AI-driven product recommendations and a 15% reduction in cart abandonment rates.
Full-service AI implementation ensures that enterprises can scale AI solutions across the organization, delivering measurable business outcomes while seamlessly integrating with existing processes. By investing in strategic planning, infrastructure, and change management, companies can overcome post-POC struggles and truly unlock the power of AI. As enterprises continue their AI journey, those that prioritize a holistic, full-service implementation approach will be best positioned to reap the long-term benefits of AI and maintain a competitive edge in their industries.
Contact us
Whether you have a request, a query, or want to work with us, use the form below to get in touch with our team.
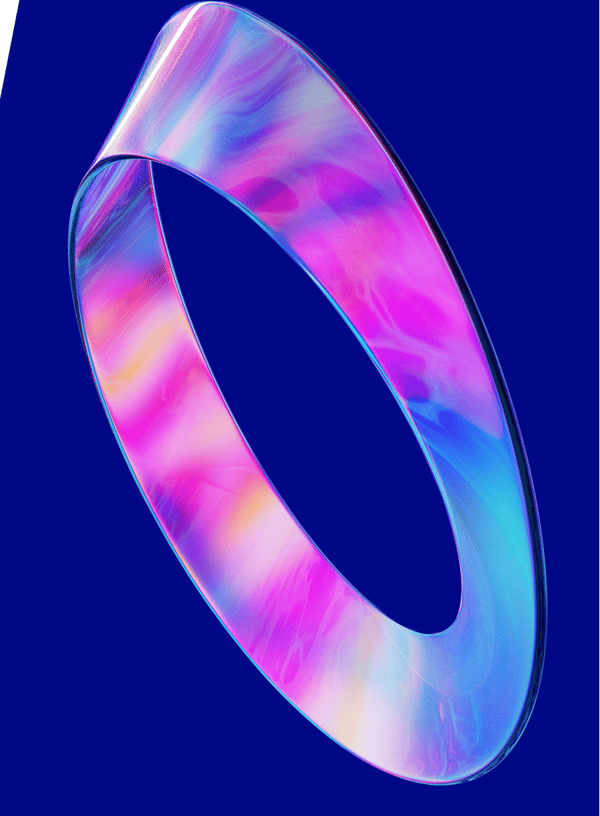

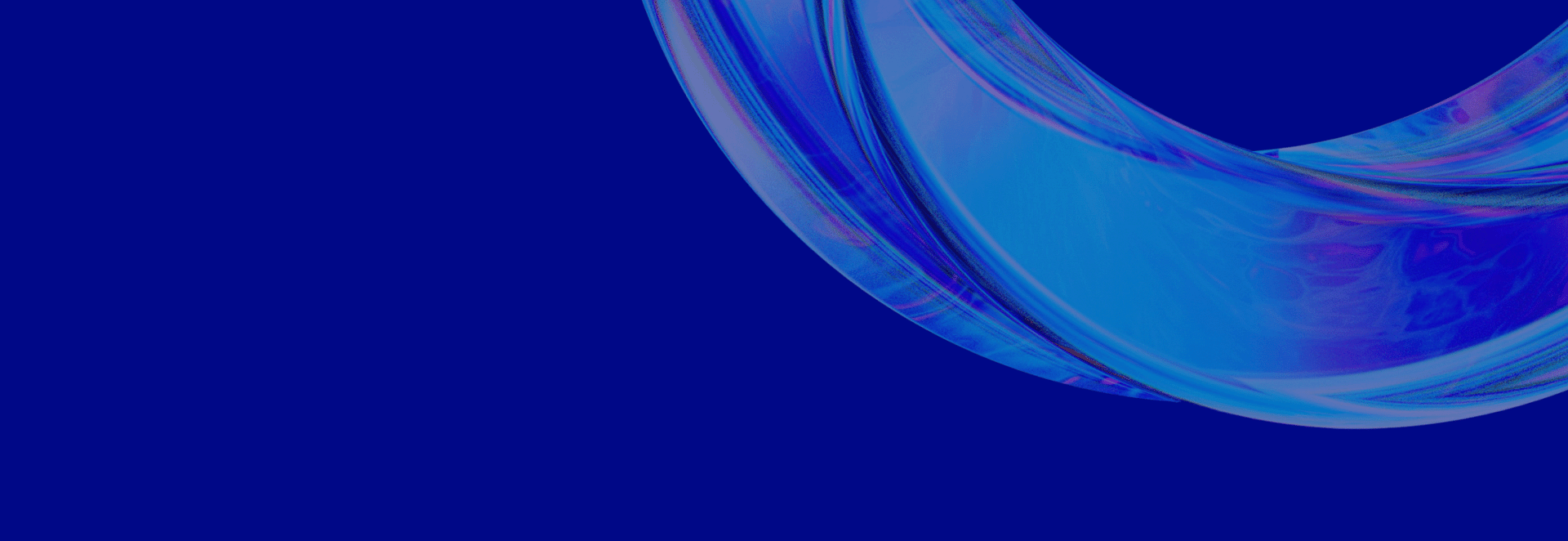
2024 Aiforce & co.
Trademark reserved

